Case Study
Soldering Fault Detection
Through the use of state-of-the-art AI algorithms, our detector identifies faults on images of solderings with a high level of accuracy, resulting in fewer false positives and false negatives compared to other similar products on the market.
The challenge
Detecting faults on images of solderings is a challenging task that requires a high level of precision and accuracy. The complexity of the soldering process, combined with the variety of potential defects that can occur, make it difficult to detect faults with traditional methods.
Moreover, small changes in lighting, orientation, or surface reflection can greatly impact the quality of the image, making it harder for human inspectors to identify faults.
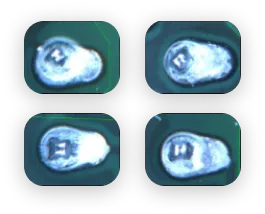
Good
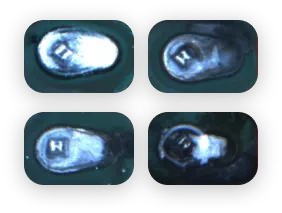
Burnt
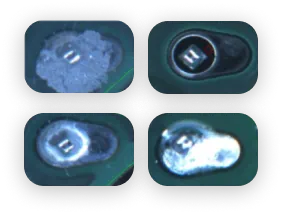
Low Paste
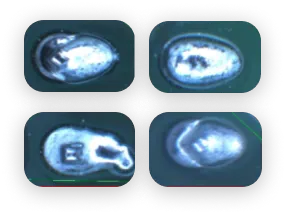
Blob
Research & Development
Throughout the project, we identified three primary types of faults. To determine the most effective approach for identifying which type the individual solderings belong to, we tested various AI algorithms, including CNN-based classification, anomaly detection, semantic segmentation, and unsupervised clustering.
Notably, unsupervised solutions proved useful in identifying previously unnoticed fault groups in addition to the previous ones.
Results
Fault detection accuracy of the Artillence AI detector outperforms the current computer vision (CV) and operator detection.
The first image array shows that the Artillence detector blocks many truly bad solder joints which were let through by the CV and operator screening. The last array shows that many good solder joints are screened out by the current system, which should be let through.
Custom Soltuions
For instances where no current camera solution is available, we are able to design and implement custom solutions. For demo purposes, we developed a custom built optical inspection cell that uses a Keyence 64MP camera, and Artillence’s advanced AI processor for detecting soldering faults during manufacturing.